Ideas in Action: An Interview with Saman Alani-Azar — AI-enabled Risk Identification Management Software Solutions
Authored by Saman Alani-Azar, Jiazhen Zhu
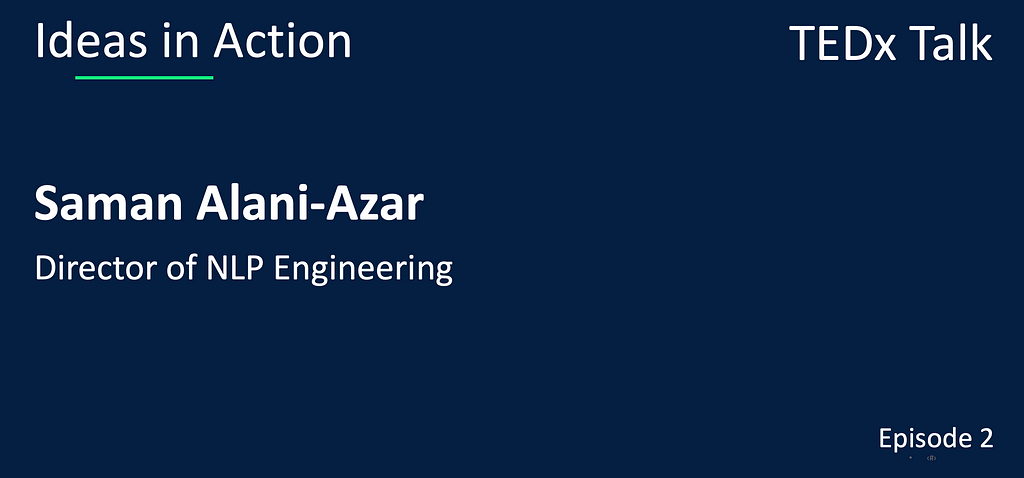
In the TEDx Talk series, Ideas in Action, we have the opportunity to engage with experts and acquire valuable insights and ideas from them. In our second installment of this series, we will be introduced to Saman Alani-Azar.
Could you briefly describe your role and responsibilities within Walmart Global Tech?
As a director of NLP engineering at Walmart Global Tech, I lead a global organization based in the United States and India. Comprising tech leaders, software engineers, machine learning engineers, and data scientists, our primary objective is to develop GenAI solutions tailored for a subset of business departments including Risk and Compliance, Global Investigation, Legal, Corporate Affairs, Sustainability, … within Walmart Enterprise Business Services.
Can you identify some common misunderstandings about data science or AI in general, and how do you typically address these?
The first common misunderstanding about AI in organizations arises at the operational level, where there’s a concern that AI might replace human jobs. This apprehension is rooted in the belief that recent AI advancements could make human involvement redundant in certain sectors. However, my viewpoint differs. I believe we are still far from being wholly replaced by machines. In fact, these advancements in AI are likely to enhance our efficiency, allowing us to focus on more complex and significant challenges within our company.
Regarding the second misconception, it’s more prevalent among leadership. Some business leaders regard AI as a universal solution, capable of resolving any issue effortlessly. There’s a common expectation that simply integrating AI will automatically solve the company’s problems. While I acknowledge the immense potential of AI as a technological solution, I also believe that in complex organizations, technology and tools must be strategically combined with the right processes, organizational culture, and people skills to ensure successful implementation.
Role of Data Scientists: How do you foresee or characterize the evolution of data scientists’ roles, particularly considering the advancement of GenAI?
The advent of GenAI is ushering in a transformative era for data scientists, reshaping their roles in profound ways. The future will likely see a reduction in the traditional coding workload, thanks to tools like GitHub Copilot, and a departure from the customary practices of building, training, and fine-tuning generic NLP models due to the capabilities of zero or few-shot LLM models. Similarly, the conventional approach to dashboard creation is being reimagined.
I think Data scientists should view this shift as an opportunity to broaden their skill sets, especially in areas like data/ML engineering and product management. By enhancing their data/ML engineering skills and leveraging cloud platform offerings for deployment, they can become more proficient at deploying their proofs of concepts and being involved in the development and deployment life cycle end-to-end.
Product management expertise equips data scientists with the ability to pinpoint and tackle the core issues that truly matter to a business, a vital capability that is frequently undervalued in numerous companies across different industries including tech. Without it, there’s a risk of chasing after the apparent symptoms of problems rather than their underlying causes. This misdirection can lead to AI teams investing time and resources on less consequential issues, which can contribute to the derailment or lack of adoption of AI initiatives and products within different organizations. Addressing this challenge calls for a close and direct collaboration between data scientists and business stakeholders to ensure a deep understanding of business needs, which can then be translated into data-driven requirements for AI products.
In addition to product management tools and methods, this process necessitates that data scientists develop soft skills, which they traditionally may not possess or prioritize since they may seem less appealing to those with a technical bent, and to effectively communicate complex data insights in a manner that is both accessible and compelling to business users who may not have a deep understanding of data science. The art of storytelling is critical. We all have heard the general (mis)-perception that “data scientists build dashboard for data scientists” implying that the dashboards are too technical and difficult to navigate and use. Or “data scientists do not demo their dashboards or results instead they are demo-lishing them” again implying lack of storytelling skills.
The other prime area where data scientists can excel is in developing robust and relevant KPIs. This involves not only selecting metrics but also deeply understanding what should be measured, its importance, how process, tech and marketing actions might move the KPIs and how it aligns with broader business objectives. They possess the expertise to identify factors impacting these KPIs and can use data to uncover these relationships. Additionally, data scientists are skilled in identifying and building the right models to improve those KPIs, whether it be optimization models, econometric models, or other analytical tools. The selection and building of these models require a level of intelligence that current GenAI tools cannot replace — at least not yet.
Another key factor to consider is the shifting expectations of our business customers regarding dashboarding and insights consumption. Users now expect to interact with our systems in a conversational manner, like how they engage with tools like ChatGPT. This necessitates a shift on our part to develop conversational systems integrated with our data warehouses. Such systems would enable business partners to pose their questions directly in plain language, without the need to navigate through multiple, potentially user-unfriendly dashboards.
Finally, it’s important to acknowledge that while GenAI is an incredibly powerful tool, it remains just that — a tool. It’s one of the many instruments in our arsenal, and its effective utilization hinges on our ability to integrate it thoughtfully within our broader strategic and operational framework. As we move forward, the value data scientists bring will increasingly lie in their capacity to leverage these tools in innovative ways to drive meaningful insights and solutions tailored to specific business needs.
Could you elaborate on your views concerning the significance of data governance and data management practices in businesses?
The importance of data governance and data management practices cannot be overstated in today’s technology-driven industries. Their relevance becomes even more pronounced in the context of GenAI and other Retrieval-Augmented Generation (RAG) based solutions, where the strength of the underlying data pipelines and ingestion modules is critical.
When we talk about the backbone of GenAI and RAG systems, it’s all about how well we handle our data. Imagine our company with tons of information scattered across different platforms like SharePoint, OneDrive, databases, and various web pages. Now, to make a RAG system work effectively, it’s crucial to bring all this info together in a streamlined way. This is where a sophisticated data pipeline comes into play.
This pipeline does more than just gather data; it’s about ensuring that every piece of information, no matter where it’s stored, is funneled into the system and kept up-to-date. Think of it like a live feed — any change or update in the original data source, be it a document or a webpage, gets instantly reflected in the system’s vector database.
Why is this so important? Well, if the RAG system is working with outdated or incomplete data, it’s like trying to solve a puzzle with missing pieces — you’re never going to get the full picture. Sure, having great prompts and safety measures against incorrect responses, like hallucination, is essential. But all those fancy guardrails won’t mean much if the core information the system relies on isn’t solid. If we get the data layer right, you’ve laid the groundwork for a system that’s not just smart, but also relevant and reliable.
At Walmart, we have been investing heavily in building the right data layers, whether they are used in our RAGs or other legal and risk applications, including e-discovery, document summarization and Q&A, media tracking for reputation risks, and e-commerce non-compliance detection, among others.
In terms of what has changed for data engineers after the GenAI boom, there are new requirements produced by conversational-based business dashboards. As I mentioned previously, business users now expect to request insights and data in plain language rather than navigating complex dashboards. This shift may lead to a new set of requirements for databases and data warehouses.
Regarding data quality and operational excellence, envision data pipelines and ingestion systems like assembly lines in automobile manufacturing. Just as meticulous quality control and operational precision are important at every stage of car production, the quality of data at each phase of a pipeline is crucial for the relevancy of the AI solutions serving specific business needs. Any lapse in this process can threaten the integrity of the entire system.
Yet, what often goes underutilized is the application of AI is monitoring the health of these data pipelines and ecosystems especially those that are very business critical. While various tests and observatory platforms, borrowed from software engineering practices, are commonly deployed to track pipeline performance, AI’s potential for anomaly detection in data flows is substantial. AI capabilities at spotting unusual customer behavior can similarly be leveraged to identify anomalies within data pipelines, such as an atypical increase in null values against historical patterns.
With respect to organizational considerations, I advocate for decentralization, placing data engineering teams within specific product teams. This structure aids in delivery speed, developing targeted and effective data pipelines, enhancing accountability, and reducing the potential for blame allocation during setbacks. Equally important is establishing processes for data discoverability and reuse to prevent inefficiency and the creation of isolated data pockets. It’s a delicate balance: while duplicate datasets are not ideal, they are a preferable alternative to the absence of data.
Questions about AI Applications in the Risk Management domain for Walmart.
When I think about AI applications for Risk Management domain of a large retail company like ours, I see a lot of similarities to my previous experience in automotive industry, where I led the AI teams for Quality, Safety, and Voice of Customers — focusing on both risk prevention and detection in service and product aspects. Despite the distinct nature of hurdles faced in the automotive field compared to those in retail and e-commerce, the foundational AI-based problem-solving framework are very similar and can be presented as follows:
- Risk Detection: The risk and compliance team requires a platform that automatically performs surveillance and compliance risk detection on various data streams. These streams are either generated or impacted by associates, sellers, buyers, and other stakeholders. Manual surveillance of every risk-related signal is often requiring considerable number of resources given the scale of Walmart and complexity of the compliance rules and regulations. To name few examples, this platform provides indications regarding products that have been recalled based on the product description or detecting different forms of fraud within our eco-systems.
- Risk measurement and Prioritization: Given the limited bandwidth of the teams, not every identified risk can be addressed and mitigated immediately. This creates a need for risk measurement and prioritization systems to rank the identified risks. The system can either use backward-looking intelligence (e.g. assessing risks based on their occurrence frequency) or forward-looking models (e.g. evaluating the potential severity if the risk remains unaddressed) to rank the detected risks for the investigation teams.
- Root Cause Analysis: This function is designed to answer “why” questions. Its primary role is to pinpoint risk amplifiers or aggravators. This is a vital step towards developing real-time preventative systems and implementing future policy changes to avoid recurring issues. For example, this system provides insights like “Sellers of type A product in region B are more likely to be incompliance relative to the other sellers.”
- Action Tracking and Feedback Loop: Once risks are identified and prioritized, and their root causes determined, it’s essential to monitor the remedial actions taken. This component provides experimentation capabilities for investigating different remedial action for a given risk and ensures that actions are tracked, measured and relevant feedback is looped back into the system. This continuous feedback helps in refining risk identification and management processes, ensuring that the system becomes more efficient over time.
This framework is versatile enough for application across various sectors, including product marketing, the financial industry, risk management, quality assurance in hardware/software, and other areas where business problem-solvers need to conduct surveillance across multiple sources to pinpoint issues or risks (e.g. as low adoption of a product feature by a subset of users where you have millions of users and variety of functionalities within the product, fraudulent financial activities, reputational risk observable in social media, and hardware part failures) that require their attention.
Finally, returning to my earlier comments on the significance of data management, in this AI-based risk management framework, having a robust, secure, and scalable data layer is even more crucial than the user interfaces and AI/GenAI technologies and algorithms employed to execute each of these four tasks. Also important to mention that adapting this framework to align with existing organizational, cultural, and process-related considerations is equally important as these other key technology and technical components.
Ideas in Action: An Interview with Saman Alani-Azar — AI-enabled Risk… was originally published in Walmart Global Tech Blog on Medium, where people are continuing the conversation by highlighting and responding to this story.