Cognitive biases can be defined as a set of predictable cognitive errors that arise from our inability to process data in an objective and balanced way. These biases frequently result from the brain’s efforts to speed up information processing. Cognitive biases can result in perceptual distortion, inaccurate judgment, illogical and improper interpretation.[1]
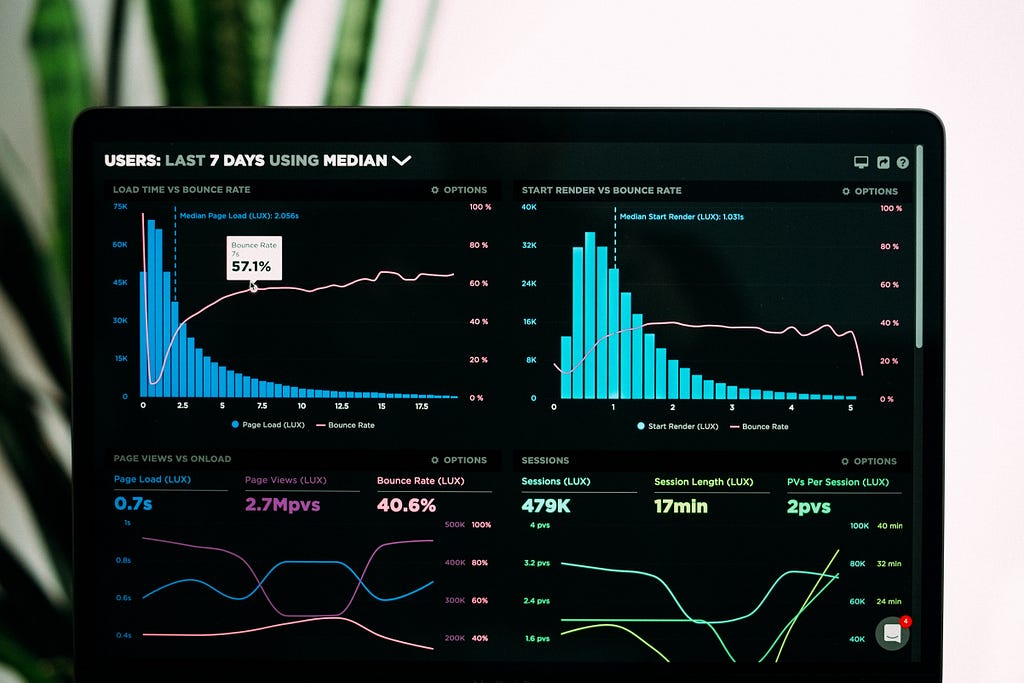
Cognitive biases have a significant impact on consumer behavior and an understanding of this phenomenon is important for any business, including e-commerce. Understanding and addressing these biases can assist e-commerce businesses in making more accurate data-driven decisions. This article will investigate some of these biases, and how to quantify and mitigate them using different techniques.
1. Anchoring bias: Anchoring bias is a cognitive bias that refers to the tendency to make decisions based on the first piece of information encountered and that serves as an anchor.
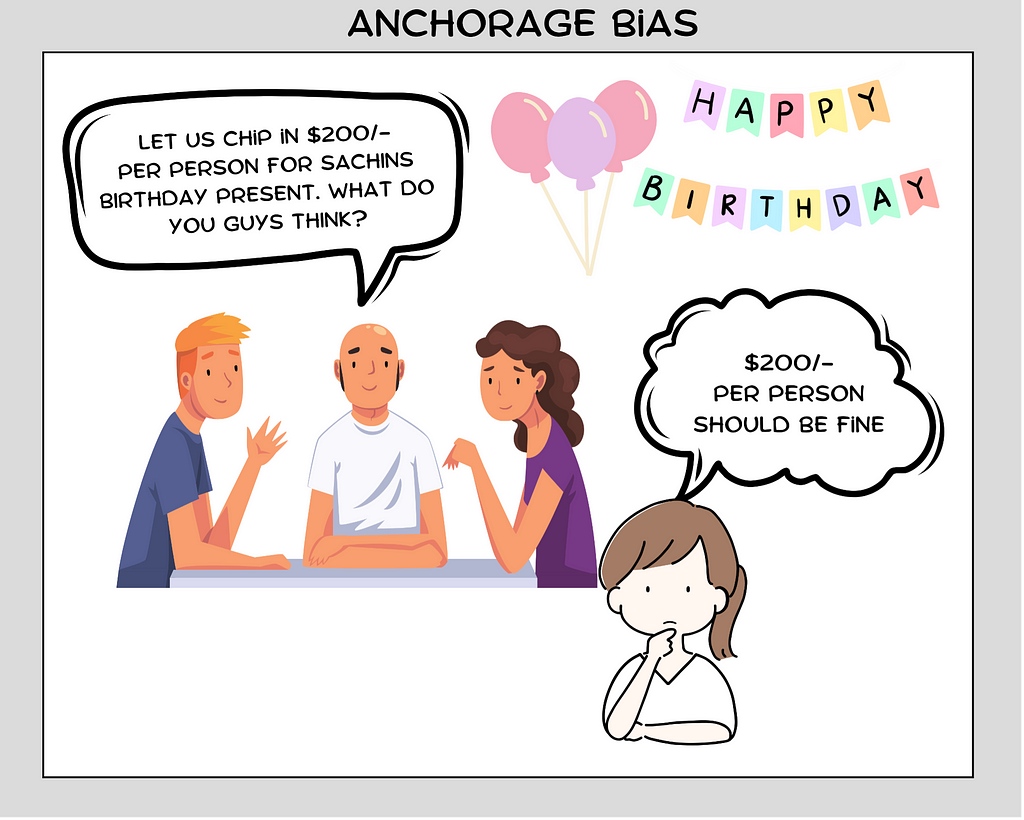
In the above example, when a friend in the group suggested a chip in of $200/- per person, this initial amount became the anchor that influenced the choices of others. If each person was asked individually about their preferred contribution, the amounts might vary significantly.
Anchoring ratio = (final estimate — anchor)/final estimate
Consider two groups A and B: A has an anchor of $40 anchor and B has an anchor of $50. Participants are then asked to select the price they are willing to pay for a better version of the product. Averages from both the groups are taken and anchoring ratio is calculated. Here the results would tell us to what extent the participants were influenced. For example, if we find that in case B the anchoring ratio is 20%, it means the participants were willing to pay 20% higher due to the anchor.
2. Confirmation bias: Confirmation bias is the tendency to seek out and interpret information that confirms preexisting beliefs.
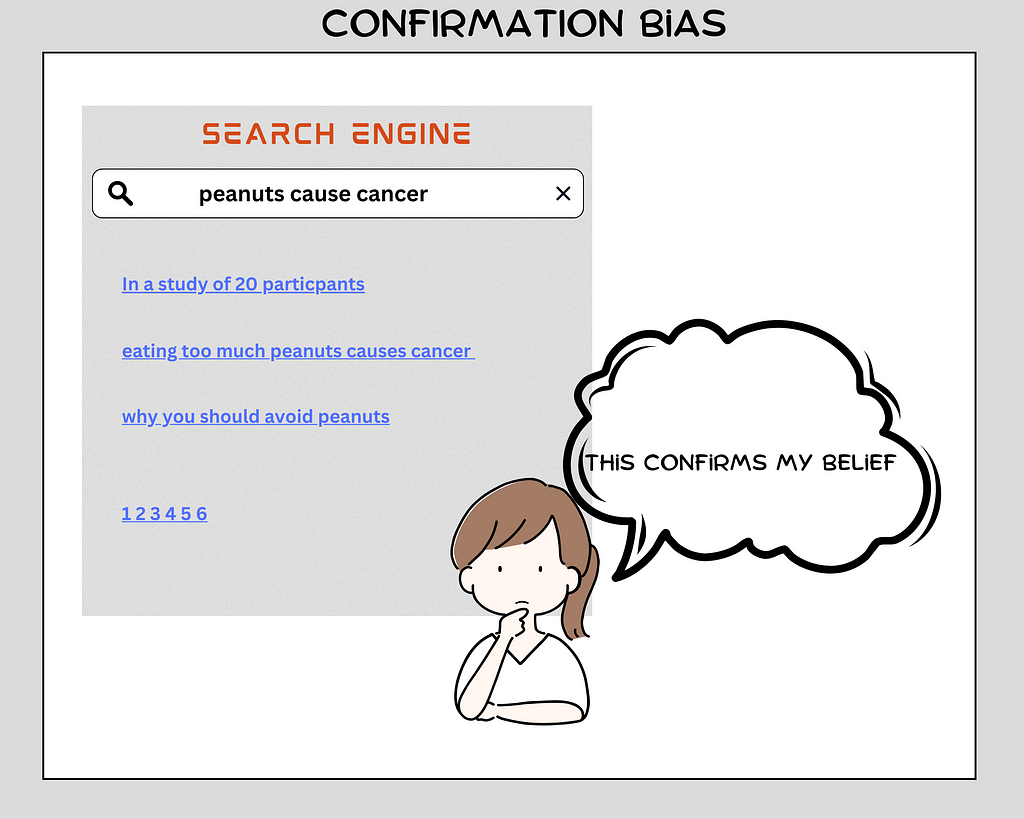
For instance, consider a product analyst working for a sports shoe company who notices a decline in sales for tennis shoes. He attributes this decline solely to a recent marketing campaign based on the numbers he finds, which indicate its lack of success. However, there could be various other factors at play, such as product quality, demand, product placement, or competitor launches. This behavior exemplifies confirmation bias, as the product analyst selectively seeks and interprets information that aligns with their preconceived belief.
To reduce confirmation bias, we can use A/B testing and effect size comparisons. A/B testing gives us data on how well each option works, helping us make decisions based on facts instead of assumptions. Effect size comparisons show how big the differences are between two groups, even when equal test/control groups aren’t possible. This helps us understand how strong the evidence is.
3. Availability heuristic: The availability heuristic is a cognitive bias that refers to the tendency to estimate the probability of an event based on how quickly relevant examples or instances come to mind or are easily available from the data.
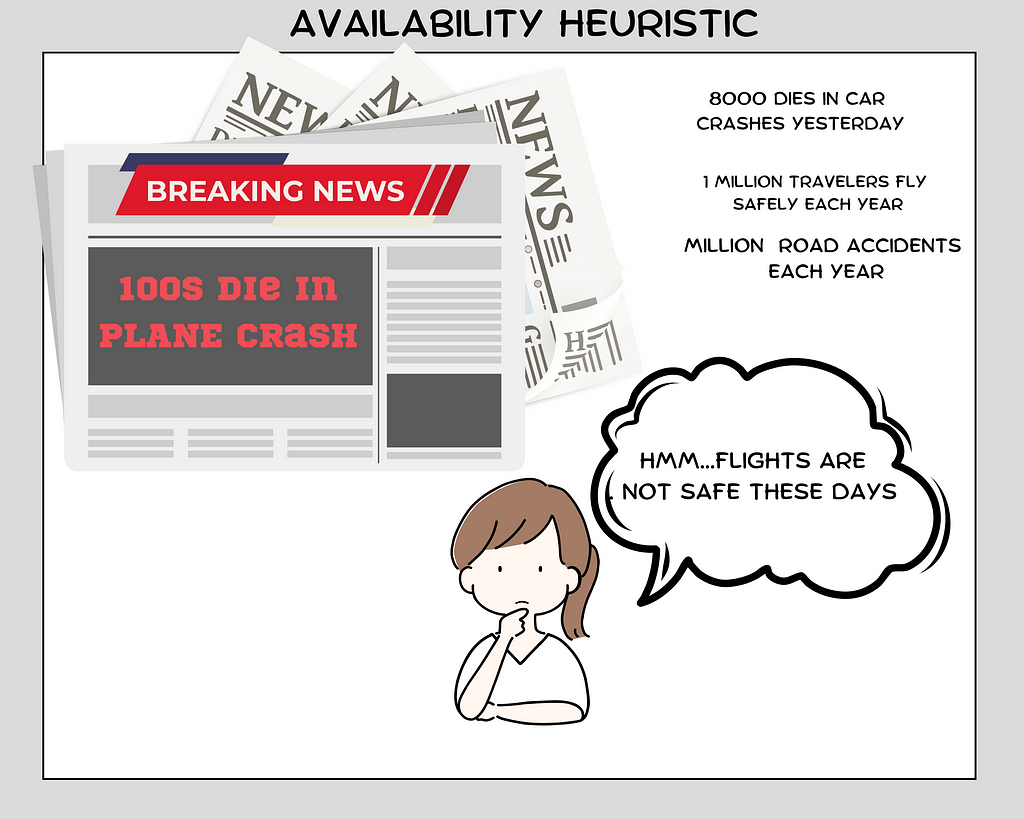
Assume that an analyst is choosing a few items to market for Search engine marketing (SEM). He has heard in several meetings that Consumer electronics products are always in top 3. Customer sales data is readily available to him. While preparing the list, he mainly selects items from consumer electronics — almost 70% of his list were consumer electronics products. Here he has fallen prey to the availability heuristic: Although the consumer electronics category always takes the first 3 positions in top sales, most of the items after that could be from APPAREL and HOME categories and those drive huge sales. He hadn’t heard about them as often because they never reach the top 3 positions.
Quantifying availability heuristic: To investigate the existence and impact of the availability heuristic, T-tests and chi-squared tests are used to compare participant estimates with the actual frequencies or probabilities of the events. Using these techniques, analysts can avoid these in real situations.
4. Status quo bias: The tendency to prefer the current situation and resist change is referred to as status quo bias. This can be seen in a variety of situations, such as decision-making, problem-solving, and risk-taking.
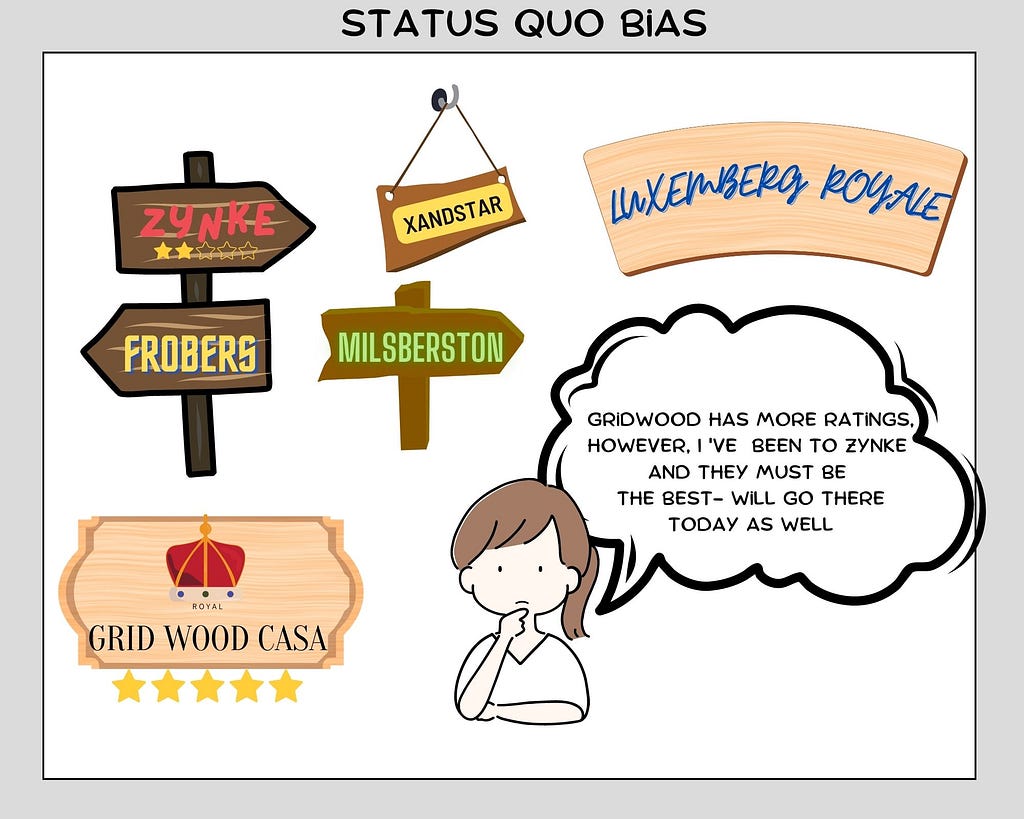
An example of status quo bias could be that a product analyst believes that assortment with good content quality will automatically always result in higher sales. The company is experiencing declining sales and has decided to improve the content quality of its assortment further. They make significant investments to improve the product images, title, description, keywords, etc. Despite investing significant resources and improving their assortment content quality, sales continue to decline. The product analysts’ belief in the relationship between better assortment content and higher sales in this scenario is an example of status quo bias. Instead, he should have considered various factors and attempted to evaluate the impact of each before proceeding with any action. This approach would have allowed for a more comprehensive and informed decision-making process.
Measurement:
Survey: A survey can be conducted to ask individuals about their level of agreement with the statement “Improving product content quality will automatically result in higher sales”. To assess the level of agreement, a Likert scale could be used.
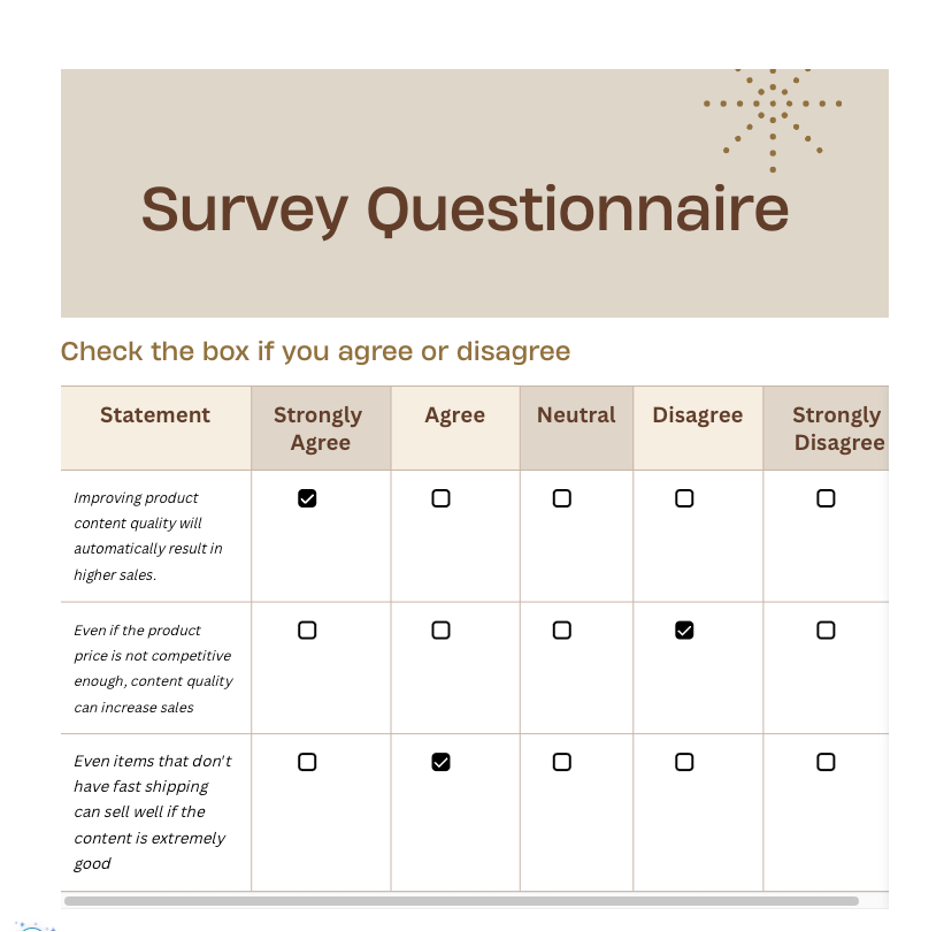
Decision-making task: To measure status quo bias, ask participants which items would have higher sales and, in the options, give few high content items as well as low-medium content items (keep changing other variables like pricing/rating/delivery). The proportion of participants who choose high-quality content as their default option can be used to assess the strength of the status quo bias.
Retrospective surveys: Retrospective surveys involve asking customers to reflect on past decisions and whether they would have made the same choice if the default option of high product quality had not been available.
We will investigate more biases and how to identify and mitigate them in the upcoming articles in this series.
References:
[1]mindtools.com/a0ozgex/cognitive-bias
[2]https://www.ncbi.nlm.nih.gov/pmc/articles/PMC3444174/
https://www.verywellmind.com/what-is-a-cognitive-bias-2794963
Predictably Irrational by Dan Ariely
Photo by Luke Chesser on Unsplash
Download this free HD photo of clearance, buying, trade and selling by Luke Chesser (@lukechesser)
https://coruzant.com/op-ed-p/mindbreeze/how-cognitive-bias-in-ai-impacts-business-outcomes/
https://vwo.com/ab-testing/
What is A/B Testing? A Practical Guide With Examples | VWO
All Illustrations created in Microsoft PowerPoint
Cognitive Biases in E-Commerce: How to Identify and Mitigate Their Influence -Part 1 was originally published in Walmart Global Tech Blog on Medium, where people are continuing the conversation by highlighting and responding to this story.